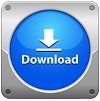
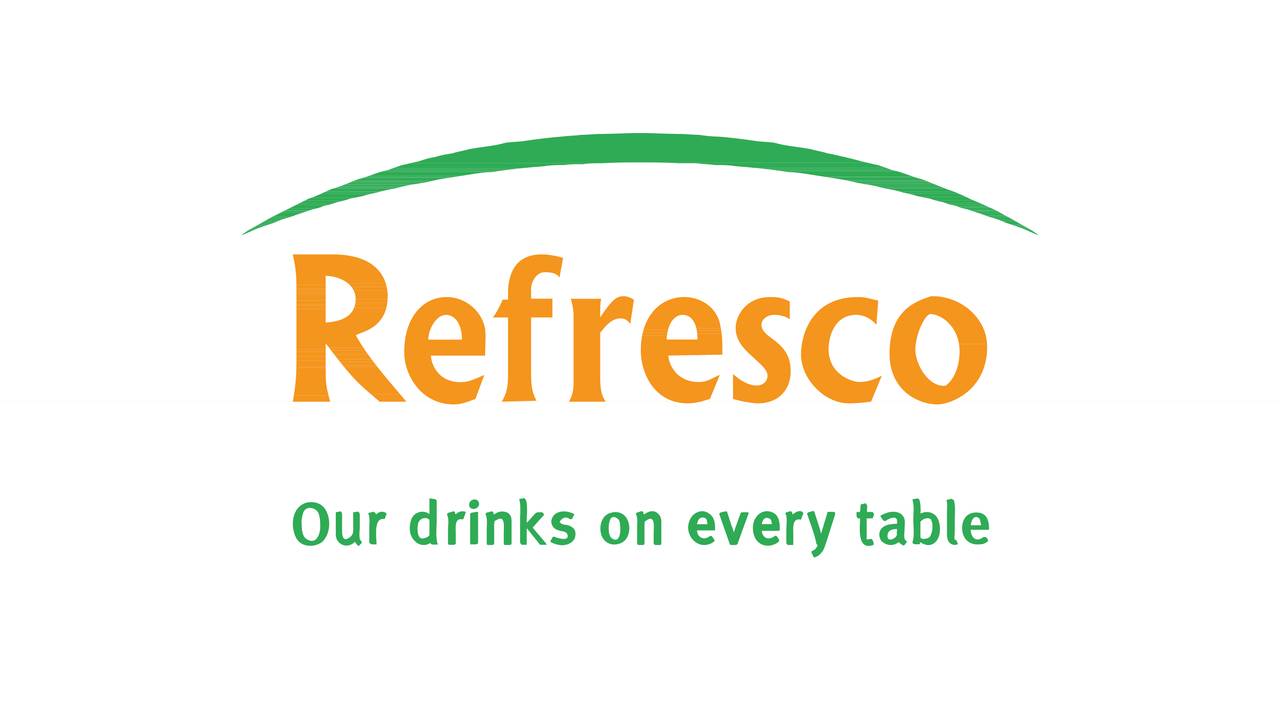
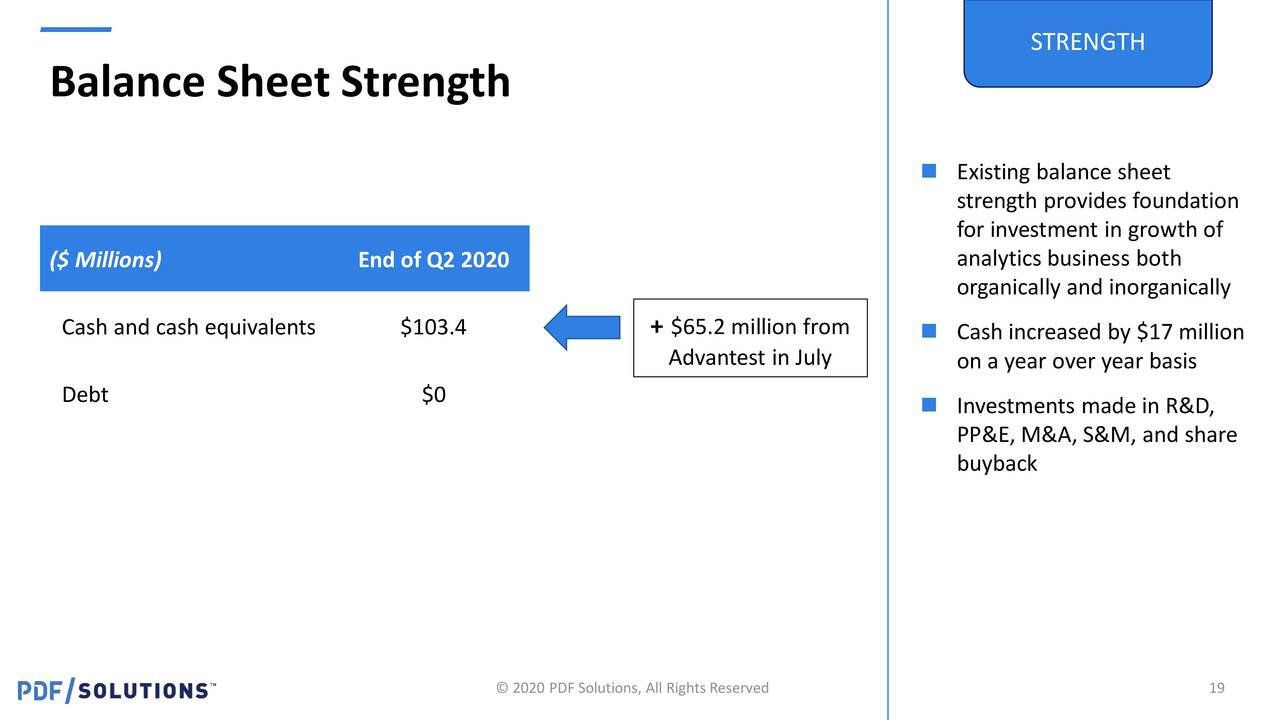
Compared to the extensive research on property heterogeneity, little effort has been devoted to studying the effect of seller heterogeneity on prices. interpretation rather challenging for market participants. Heterogeneous seller behavior, strategies, and decisions cause observable transaction prices to be "noisy" in the sense that the observed data may be biased indicators of the underlying property values or market conditions.
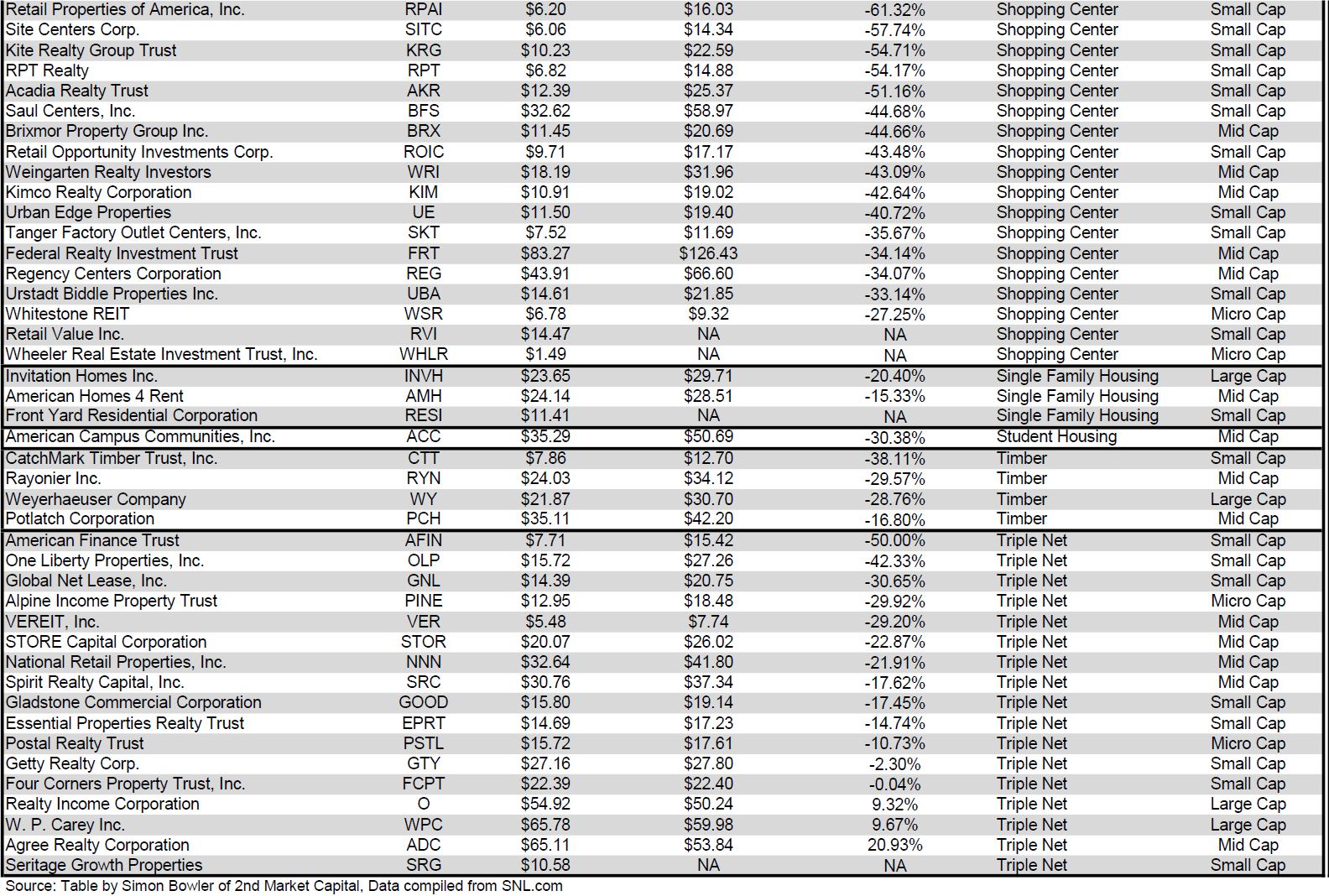
This paper provides a theoretical framework to examine how the differences in seller motivation can potentially affect the distribution of home prices. The evidence suggests an interesting alternative to agent performance: that buyers and sellers with different reservation price and time on market expectations, such as those selling foreclosure homes, tend to select agents along gender lines. Clear differences in real estate sales prices, time on market, and agent incomes across genders are unlikely due to differences in negotiation performance between genders or the mix of genders in a two-agent negotiation. Although agent gender seems to play a role in naïve models, its role becomes inconclusive as variables controlling for possible price and time on market expectations of the buyers and sellers are introduced to the models. A sample of 2,020 home sales transactions from Fulton County, Georgia are analyzed in a two-stage least squares, geospatial autoregressive corrected, semi-log hedonic model to test for gender and gender selection effects. This study examines differences in net selling price for residential real estate across male and female agents. Copyright 2008 American Real Estate and Urban Economics Association These findings can help to explain the apparent "risk-premium puzzle" in real estate. In addition, we find that, given the typical 5- to 7-year holding period for real estate, the Sharpe ratios estimated by integrating both price and TOM risk are much in line with the performance of financial assets. Third, our empirical applications in both residential and commercial real estate markets show that the Sharpe ratio estimated by the traditional approach is seriously overstated-to the largest extent for investors with high financial distress. Second, the traditional valuation of real estate return and risk, which is based solely on the return distribution of a successful sale without considering the uncertainty of TOM and the investor's financial circumstances, underestimates real estate risk and exaggerates real estate return. First, we show that real estate return and risk, which account for both price and TOM risk, are investor specific, varying over investors with different financial circumstances and holding periods. The major findings of this article can be summarized as follows. We demonstrate that real estate sellers with different degrees of financial distress face not only different marketing period risks, but also receive different return distributions upon successful sales. This article develops a theoretical framework and formulates a unified risk metric that integrates both real estate price risk and uncertainty of time on market (TOM).
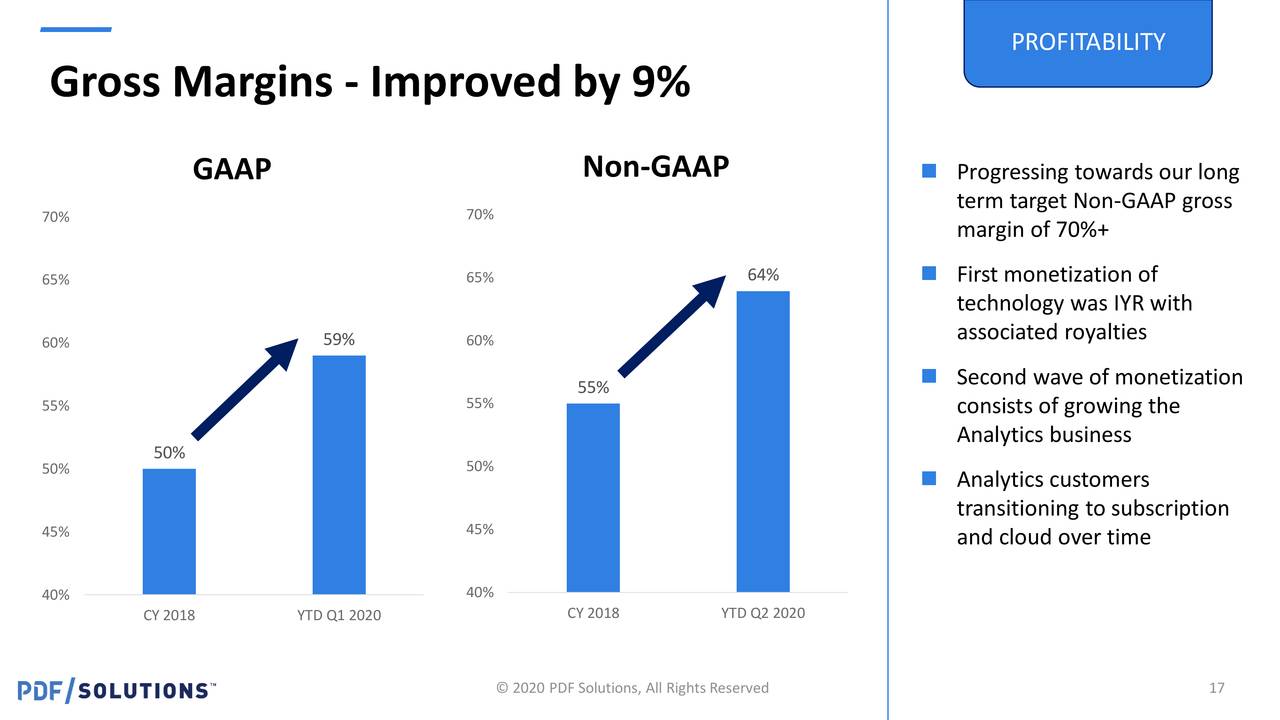
Each subsample is further divided into three subsamples based on if the resale occurred during “Bubble” (January 2000 to June 2007), “Crisis” (July 2007 to September 2009) or “Rebound” (October 2009 to December 2013) Among all resales via regular methods (excluding REOs and short sales), “Normal flips” is a subsample that with houses resold within 2-Year from Purchases (excluding same-month resales), “Same-month flips” is a subsample that with houses resold within the same-month of purchases, and “Non-flips” is a subsample that with houses resold after 2 years of purchases. The explanatory variables in the pricing regressions include house age, living space, land size, 1 to 5 bedroom number dummies, 1 to 3 full-bathroom number dummies, 1 to 2 half bathroom number dummies, 1 to 2 story number dummies, dummies for top 10 zipcodes with most frequent resales, quarter dummies, and so on. Using the data of one of the most frequent MSAs in our sample, Chicago-Joliet-Naperville, we estimate the expected purchase price, listing price and sale price from their pricing regressions, and then calculate the Degree of Over-Pricings for purchase price DOPb, for listing price DOPl, and for sale price DOPs, as their percent differences from their expected prices. Degree of Over-Pricing for Resales in Chicago-Joliet-Naperville.
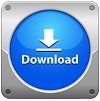